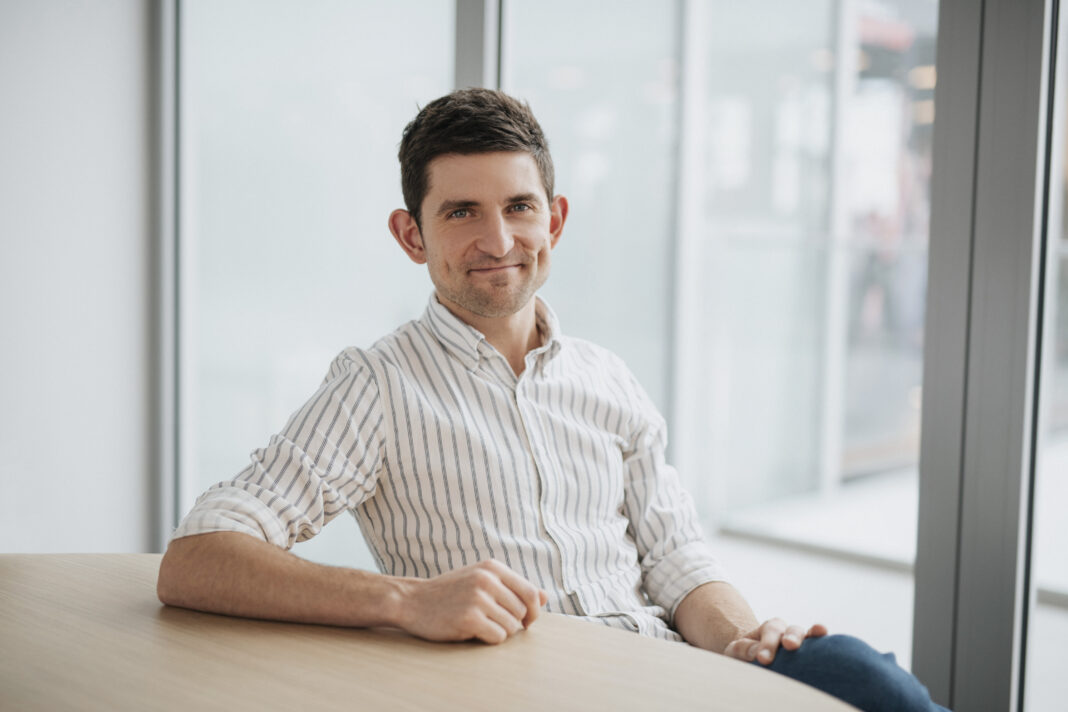
Piotr Orzechowski, Co-Founder and CEO of Infermedica
Innovation areas to watch: One of the exciting areas of applied machine learning recently is so-called Generative Tech. Some say it’s the biggest change to the Internet since crypto was invented. Generative Tech is developing at an unprecedented pace and many of us already had a chance to enjoy images created by OpenAI’s DALLE-E or content generated by GPT-3 language model. However real-world applications of generative tech in health are either scarce or non-existent. It would be exciting and somewhat unexpected to see this technology enter the market in 2023 with language models trained specifically on medical literature or patient records. Obviously a myriad of challenges occurs in such an approach – starting from regulatory approval, to clinical validation, preventing bias and defining the intent of use – but I see Generative Tech as an engine potentially powering NLP-based chatbots and virtual assistants.
Sandeep Shah, CEO and Founder of Skyscape
AI is ready for disruption in healthcare, beginning to assist providers who are struggling to do more with less. With a rising inflation rate, staff shortages across all aspects of healthcare, and the compounding effect of “doing more with less,” healthcare companies must turn to technology to simplify their workloads.
With large volumes of data and AI entering healthcare, providers can simplify administrative tasks by outsourcing to AI-powered automation. The biggest criticism of AI is its dehumanizing aspect, but with careful and cultural considerations, AI can free clinicians from the menial, repetitive administrative tasks that enable them to focus their attention more on patient care.
Non-human resources such as facilities, beds, or OR rooms will be more intelligently leveraged with better scheduling and utilization. This is especially crucial in the post-Covid surge in the preventative and chronic disease care that was artificially suppressed in the last 2+ years.
Further experimental services with AI could provide more reliable medical content, as opposed to existing misinformation available via Google or search engines. Patient care questions can be more carefully vetted with appropriate source content libraries.
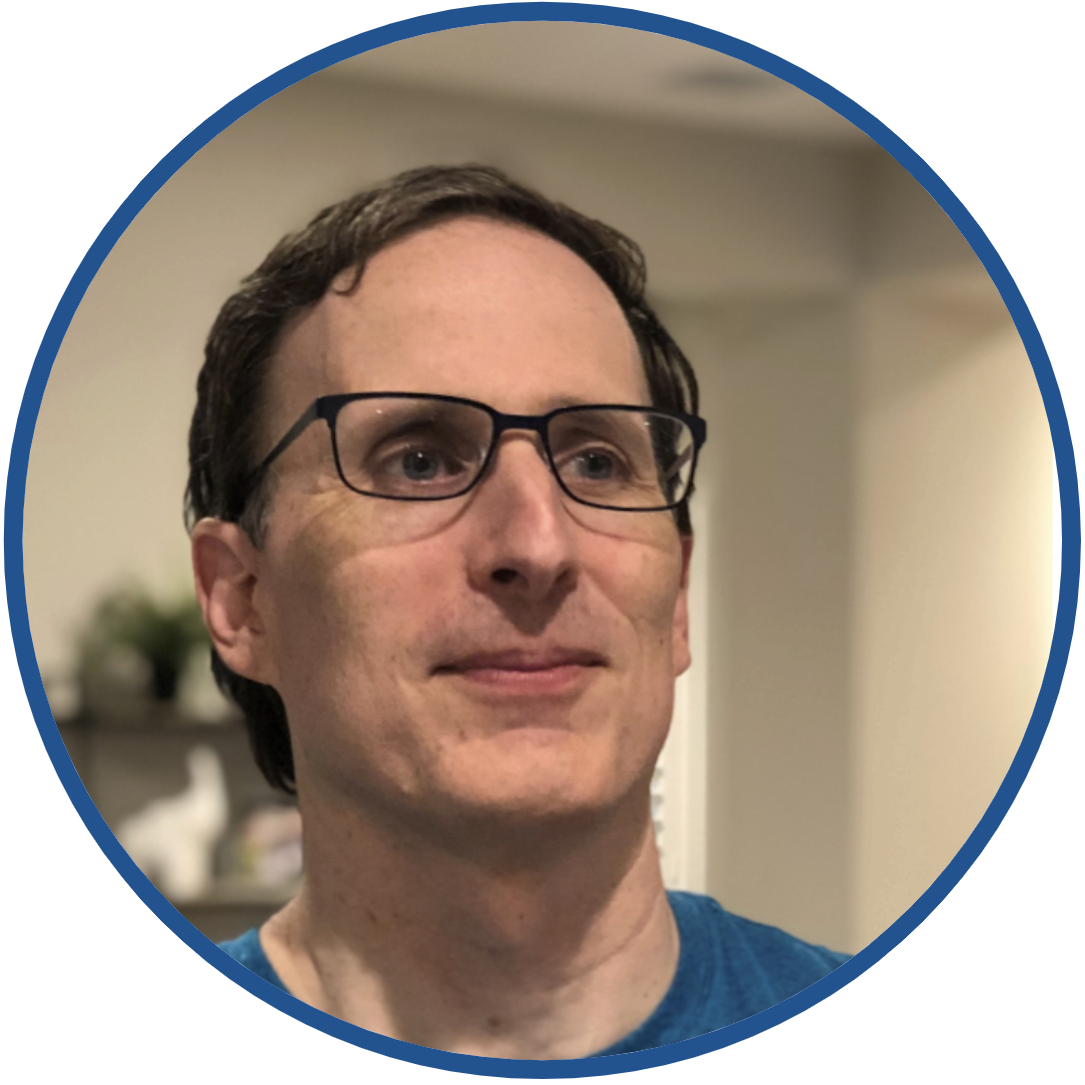
Michael Elashoff, CEO and co-founder of Cornerstone AI
Automation and ML will increasingly handle the work of data preparation in health data science, leading to layoff pressures across the industry. Data scientists and teams with strong healthcare expertise will weather this storm by focusing on fusing human insight with AI models in novel ways. This includes innovations in interpretable AI that give biological and clinical explanations to model predictions and new methods to fill in the gaps in medical records with clinically appropriate values to enable broader accessibility of AI algorithms.
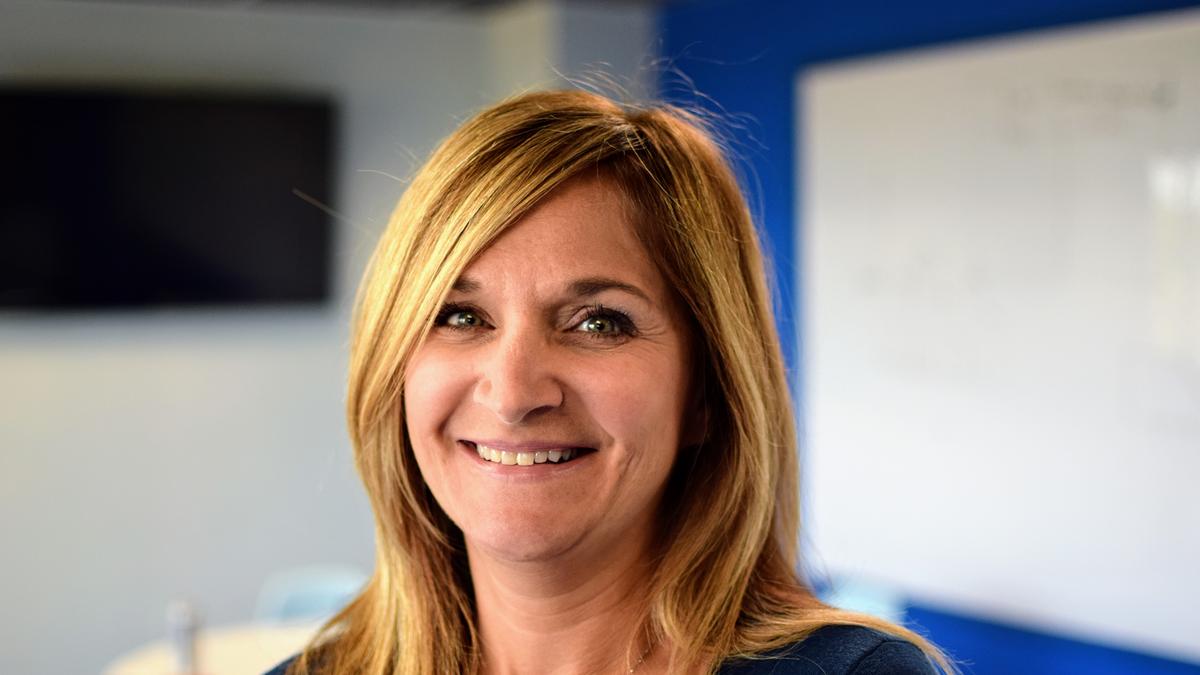
Joan Butters, CEO and Co-Founder, XSOLIS
In the coming year, AI will become even more integrated into the healthcare system, across all sectors, as the clinician shortage worsens. To address the growing staffing crisis and lingering revenue challenges, financial and operational executives will increasingly realize and pursue the immediate benefits that AI solutions can lend to stop the hemorrhaging in both of these areas – enabling staff to create efficiencies while improving the bottom line. In the spirit of delivering on how to do more with less, the use of technological solutions to meet these goals will greatly accelerate to reduce administrative tasks, friction and waste, to ensure the appropriate patient care in the right setting, at the right time, while reducing the cost of care.
Ittai Dayan, CEO of Rhino Health
AI has great promise in both clinical and non-clinical use cases. AI applications have yet to achieve their full potential as of 2022, however. A critical limitation is the lack of access to the diverse, high-quality data needed to train and maintain models. Because of this lack of data access, developers often resort to creating algorithms with data from a single site. The resulting models are often not “generalizable” and do not perform well when introduced to data dissimilar from the narrow training set. The models may not only perform poorly, limiting efficacy and impact, but may also contain bias. Algorithmic bias propagates the underlying data bias and may result in less effective AI products. This negative feedback loop then results in lower adoption.

Dave Hodgson, CEO & Co-Founder at Project Ronin
We anticipate the market for artificial intelligence in healthcare will grow geometrically in the coming years. However, this pace will be strongly guided by the ability of solution vendors to demonstrate safe, ethical, and clinically appropriate use. The paramount challenge to healthcare AI in 2023 will be building credibility among impacted stakeholders–clinicians applying AI at the point of care, administrators paying for AI solutions, patients offering data for improved care, and more. In addition to ensuring that we have evidence of impact on outcomes, satisfaction, and cost, 2023 will bring more distinction and definition around healthcare AI and machine learning.
Itay Klaz, MD, MHCI, FACHE, Medical Director for Clinical Surveillance & Compliance at Wolters Kluwer
AI has great promise in both clinical and non-clinical use cases. AI applications have yet to achieve their full potential as of 2022, however. A critical limitation is the lack of access to the diverse, high-quality data needed to train and maintain models. Because of this lack of data access, developers often resort to creating algorithms with data from a single site. The resulting models are often not “generalizable” and do not perform well when introduced to data dissimilar from the narrow training set. The models may not only perform poorly, limiting efficacy and impact, but may also contain bias. Algorithmic bias propagates the underlying data bias and may result in less effective AI products. This negative feedback loop then results in lower adoption.

Tim Kostner, Chief Revenue Officer, XSOLIS
In 2023 we will continue to see a push to realize value in healthcare through the use of data and automation. Specifically, the increasing awareness of the fragmentation that exists across the traditional payer-provider model, will result in initiatives that foster a more collaborative approach. In addition to more robust clinical data-sharing, we will see early examples of how advanced automation and AI models can be leveraged to create efficiencies and a more coordinated, patient-centric model. Simply, given the workforce, capacity and economic challenges faced, the environment is ripe for disruption.
Marie Lamont, Global RWE Data Strategy, Access & Enablement at IQVIA and General Manager at Inteliquet
AI technology is assisting in matching patients with new therapeutics appropriate to them. It removes the human biases to normalize, confirm and present patients that are a match for clinical trials. AI technology can provide a very high degree of sensitivity and specificity, which offers a more complete picture about a patient. Additionally, these technologies assist in automating data source verification in trials. In 2023, we envision AI technology use will further streamline the clinical trial research market through helping to quickly identify the right patient for the right trial and drive greater data efficiencies. Partnering with healthcare institutions to drive AI for clinical trials and patient care delivery is key.